20 Good Facts For Choosing Coincheckup
Top 10 Tips To Profit From Sentiment Analysis In Ai Stock Trading, From The Penny To The copyrightThe use of sentiment analysis within AI trading stocks can be a great method of gaining insights into the market's behavior, particularly for penny stocks and cryptocurrencies where sentiment plays an important part. Here are ten top suggestions for effectively using sentiment analysis for these markets:
1. Sentiment Analysis What exactly is it and why is it significant?
TIP: Be aware of the fact that short-term movements in prices are influenced by sentiment especially on speculative stocks and copyright markets.
Why: The public's sentiment is usually a key indicator of price movement which is a great signal to trade.
2. Use AI to analyze a variety of Data Sources
Tip: Incorporate diverse data sources, including:
News headlines
Social media: Twitter, Reddit Telegram and other social media.
Blogs and forums
Earnings calls Press releases, earnings announcements
The reason: Wider coverage gives a greater sense of completeness.
3. Monitor Social Media In Real Time
Tip : You can follow trending conversations using AI tools, such as Sentiment.io.
For copyright Concentrate on influencers.
For Penny Stocks: Monitor niche forums like r/pennystocks.
Why is this? Real-time tracking lets you to capitalize on emerging trends.
4. The focus is on the Sentiment Metrics
TIP: Pay attention to indicators like:
Sentiment Score: Aggregates positive vs. negative mentions.
It tracks the buzz or excitement around an asset.
Emotion Analysis: Assesses anxiety, fear or uncertainty.
What are they? These metrics provide real-time insight into the psychology of markets.
5. Detect Market Turning Points
Tips: Make use of data on the sentiment of people to find extremes in positive and negative.
Contrarian strategies thrive in extreme circumstances.
6. Combining Sentiment and Technical Indicates
Tips: Check for sentiment using standard indicators, like RSI, MACD or Bollinger Bands.
The reason: Sentiment isn't enough to give context. an analysis of the technical aspects can be useful.
7. Automated Sentiment Data Integration
Tip: Use AI trading bots, which incorporate sentiment scores into their decision-making algorithms.
The reason: Automated market reaction permits quick responses to changes in sentiment.
8. Account for Sentiment Management
TIP: Beware of pump-and-dump schemes and fake news, particularly with copyright and penny stocks.
How: Use AI software to identify anomalies.
What is the benefit of recognizing manipulators and avoiding false signals.
9. Backtest Sentiment-Based Strategies
Tip: Test the way sentiment-driven trades been performing in previous market conditions.
Why: You can use sentiment analysis to help improve the strategies you employ to trade.
10. Tracking the sentiment of key influencers
Tip: Make use of AI to track market influencers like prominent traders, analysts or copyright developers.
Focus on the tweets and posts of people like Elon Musk or any other blockchain-related founders who are prominent.
Keep an eye on industry analysts and activists for Penny Stocks.
Why: Influencer opinions can greatly influence the market's opinions.
Bonus: Combine sentiment with basic data and data from on-chain
Tip: Integrate sentiment with the fundamentals (like earnings reports) for penny stocks as well as on-chain data (like the movements of wallets) for copyright.
Why: Combining different kinds of data provides a more holistic view, and less emphasis is placed on sentiment.
With these strategies to implement these tips, you can leverage sentiment analysis in your AI trading strategies for penny stocks and copyright. Follow the best redirected here for ai trading platform for site examples including best copyright prediction site, best copyright prediction site, ai investing app, best ai penny stocks, using ai to trade stocks, trade ai, ai stock trading app, ai stock analysis, ai copyright trading bot, ai stock analysis and more.

Top 10 Tips For Profiting From Ai Stock Pickers, Predictions, And Investments
Backtesting tools is critical to improving AI stock selection. Backtesting allows AI-driven strategies to be tested under previous market conditions. This gives insights into the effectiveness of their plan. Backtesting is an excellent tool for stock pickers using AI as well as investment forecasts and other instruments. Here are ten tips to help you get the most benefit from backtesting.
1. Use High-Quality Historical Data
Tips: Ensure that the software you are using for backtesting uses comprehensive and accurate historical information. This includes the price of stocks and dividends, trading volume, earnings reports as well as macroeconomic indicators.
Why: High-quality data ensures that the backtest results are accurate to market conditions. Incomplete data or incorrect data can lead to inaccurate backtesting results that can affect your strategy's credibility.
2. Include realistic trading costs and slippage
Tips: When testing back make sure you simulate real-world trading costs, such as commissions and transaction costs. Also, think about slippages.
The reason: Not accounting for trading and slippage costs can lead to an overestimation of the potential return from your AI model. These variables will ensure that the backtest results are in line with real-world trading scenarios.
3. Tests across Different Market Situations
Tip back-testing the AI Stock picker to multiple market conditions, such as bull markets or bear markets. Also, you should include periods that are volatile (e.g. a financial crisis or market correction).
The reason: AI-based models could behave differently in different markets. Testing in various conditions assures that your plan is robust and able to change with market cycles.
4. Test with Walk-Forward
Tip Implement walk-forward test, that tests the model by evaluating it using a a sliding window of historical information, and then comparing the model's performance to data that are not in the sample.
What is the reason? Walk-forward testing lets you to test the predictive power of AI algorithms on unobserved data. This is an extremely accurate method to assess the real-world performance opposed to static backtesting.
5. Ensure Proper Overfitting Prevention
Beware of overfitting the model through testing it on different times. Also, make sure the model isn't able to detect the source of noise or anomalies from historical data.
Overfitting occurs when a model is tailored too tightly to historical data. It becomes less effective to predict future market movements. A well-balanced model must be able to generalize across different market conditions.
6. Optimize Parameters During Backtesting
TIP: Backtesting is great way to optimize important parameters, such as moving averages, positions sizes and stop-loss limit, by repeatedly adjusting these parameters before evaluating their effect on returns.
The reason: Optimizing these parameters can enhance the AI model's performance. As mentioned previously, it is important to ensure that this optimization will not lead to overfitting.
7. Drawdown Analysis and risk management should be a part of the overall risk management
Tip: Include risk control techniques including stop losses Risk to reward ratios, and positions sizing when backtesting to assess the strategy's resistance against large drawdowns.
The reason: Proper management of risk is essential for long-term success. Through simulating how your AI model does when it comes to risk, you are able to spot weaknesses and modify the strategies to achieve better risk adjusted returns.
8. Determine key metrics, beyond return
Tips: Concentrate on the most important performance metrics beyond simple returns like the Sharpe ratio, the maximum drawdown, win/loss, and volatility.
These indicators can help you comprehend the AI strategy's risk-adjusted results. If you solely rely on returns, you could overlook periods of significant volatility or high risk.
9. Simulation of various asset classes and strategies
TIP: Test the AI model with different types of assets (e.g. ETFs, stocks and copyright) as well as various investing strategies (e.g. mean-reversion, momentum or value investing).
Why: Diversifying backtests across different asset classes lets you to evaluate the adaptability of your AI model. This ensures that it is able to be utilized across a range of markets and investment styles. It also helps the AI model to work with high-risk investments like cryptocurrencies.
10. Refine and update your backtesting method often
Tips: Make sure to update your backtesting framework on a regular basis with the most recent market data to ensure it is up-to-date to reflect the latest AI features as well as changing market conditions.
Why Markets are dynamic as should your backtesting. Regular updates make sure that your backtest results are accurate and that the AI model is still effective when new data or market shifts occur.
Bonus: Monte Carlo Simulations are helpful in risk assessment
Tips: Monte Carlo simulations can be used to simulate different outcomes. Perform several simulations using various input scenarios.
Why: Monte Carlo Simulations can help you determine the probability of a variety of outcomes. This is particularly helpful when dealing with volatile markets, such as copyright.
If you follow these guidelines using these tips, you can utilize backtesting tools effectively to assess and improve the performance of your AI stock picker. By backtesting your AI investment strategies, you can ensure that they are robust, reliable and able to change. Read the most popular free ai trading bot for blog advice including stock ai, ai trading bot, free ai tool for stock market india, ai penny stocks to buy, coincheckup, ai financial advisor, ai penny stocks to buy, ai penny stocks to buy, ai investing, trading ai and more.
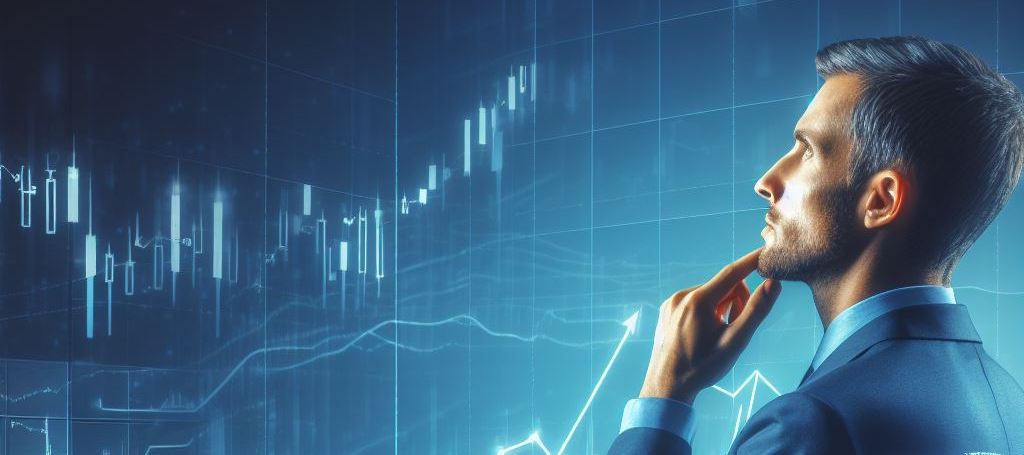